How to Become a Data Scientist If You Lacking the Necessary Skills
Embrace Change, Love Learning, and Persist
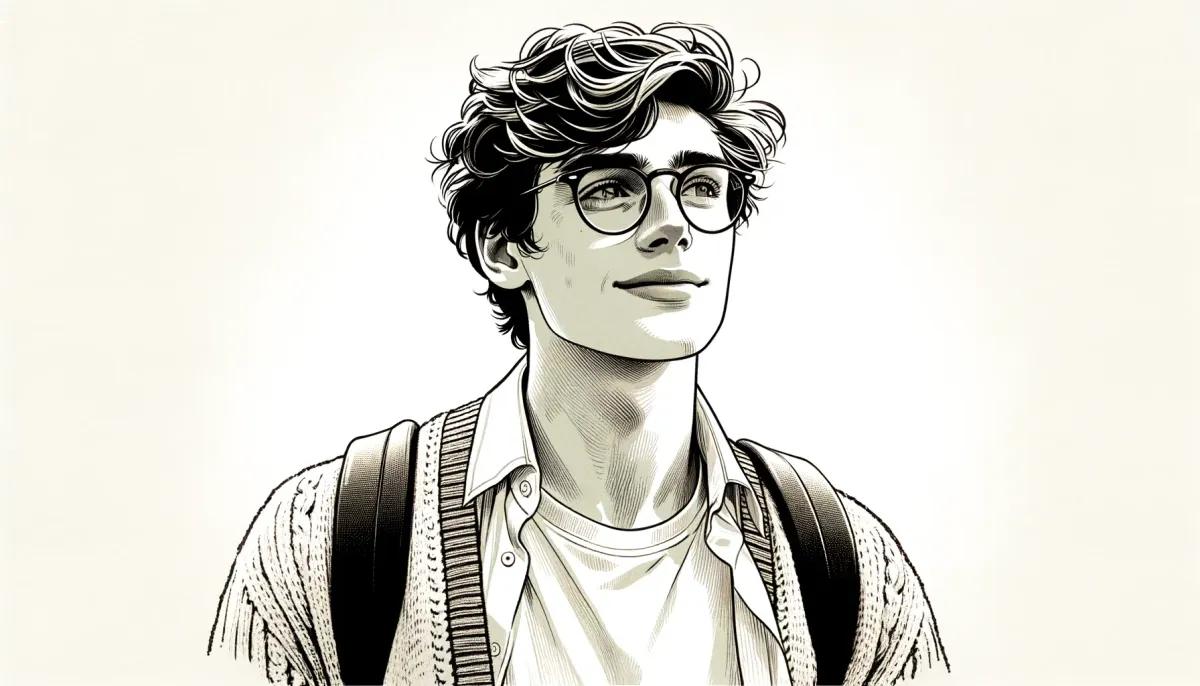
Recently, during a lecture for campus recruitment, a student asked me: He only knows math and basic sciences but doesn't know how to code. Can he still become a data scientist?
First off, yes, absolutely.
How to do it? First, understand if becoming a data scientist is your goal or a part of your journey. From what I see, you're asking how to achieve this goal.
That involves figuring out what skills you need and which paths to take.
Start Working Hard Now
No one understands all knowledge areas from the start. Generally, school education lays a foundation for your career but that doesn't mean your learning stops at graduation.
Academia often lags behind market demands. We can't all start with the skills needed for a job. Even if we have them, they're just formulas and theories from books, far from what companies actually need.
Take me, for example. I've been in the big data field for nearly 15 years, working as a senior data scientist at a giant internet company, and now I'm the chief data architect at a large brokerage firm's big data department.
But you know what? When I was about to graduate, I was just an undergrad in business administration from an ordinary university.
Unlike you, I started with some programming experience. I had taken courses in linear algebra and calculus, but at the time, I didn't see the point in learning these theories. My first challenge was finding a decent job, not thinking about a long-term goal like data science.
So, I took stock of my strengths and available tools. I loved programming; I had plenty of time, unlike now, and I could access the school's library and computers for free, giving me a low-cost learning opportunity.
While my dorm mates were lost in World of Warcraft, I spent two hours every day immersed in programming. I mastered basic design patterns and algorithms and even got a Java certification.
Also, I'm an introvert, or a nerd as you might say, not keen on talking much. So, I forced myself to join student council activities and interact with opinion leaders or join some speeches to develop my ability to express myself.
Looking back, if I could do college over, I'd take more internships to better understand what companies were doing to prepare faster.
Is it tiring? Yes, but we have no choice. Once we set a "big" goal, we must keep going. Forced persistence won't work; you must also love what you do.
Keep the Love, Stay Focused
Common wisdom says when you graduate, you're a blank slate with endless possibilities and many potential paths. But why choose to be a data scientist? Apart from the recent boom in big AI models, maybe because you love the field.
Love is the original driving force that keeps a person growing and persisting.
Why did I want to be in programming?
In college, I deeply considered my future. Most from my business admin program went into real estate sales or maybe design institutes if they were more skilled.
Could I handle those jobs? The thought of dealing daily with clients or attending lengthy lunches made me feel unfit.
What could I do? I remember programming in BASIC in elementary school, writing scripts in Flash during high school, and doing well in college programming courses.
Though these were mere toys compared to enterprise programming, I genuinely enjoyed sitting at a computer with reference docs all afternoon and not getting bored. I think that was proof of my love for the field.
So why consider other possibilities? I decided to stick with programming and have been in the field ever since, despite temptations and confusion along the way.
The 10,000-hour rule is real. If you keep loving and pursuing this field, you'll become an expert.
Okay, I've said a lot that seems unrelated to data science. Can we get back on topic?
Data science wasn't a job when I started working; the closest was "data mining engineer." As companies grew and technology advanced, data science emerged.
Embrace Change
You never cross the same river twice. Likewise, business logic won't wait for you to get ready.
To stay competitive, you must continually adapt your skills to meet talent demands. I'm not an HR expert, but I'll share how I grew into a data scientist.
After graduating, thanks to my schooling, I joined a giant company's data mining team as a junior developer.
Initially, I did data development. As the team grew, we needed Java engineers for web apps, and I was chosen despite my reluctance since I was just getting comfortable with SQL.
But this change was a blessing. Working as a Java engineer let me apply the design patterns and object-oriented programming I learned in college. Being closer to business in app development helped me understand end-user pain points, aiding my career growth.
Over the years, I switched roles as needed: from enhancing user experience as a front-end developer to building data platforms back in data development, and managing recommendation models as part of MLOps, eventually becoming a data science expert.
Some ask, didn't you say to stay focused? Why change roles?
I don't see a contradiction. When you extend your career over decades, it's hard to stick to one niche. By exploring different roles, I understood what companies needed from an engineer. I stayed in big data, and my diverse background helped me communicate and collaborate across roles.
But no matter what, you're working to earn your salary. How do you excel in these various roles? My answer is continuous learning.
Continuous learning
Initially, I had basic Java skills, but my first job was writing SQL.
Imagine my excitement and fear when I got the offer. I couldn't afford to lose this job, so I spent a week learning Oracle database development, sleeping only a few hours a night. I don't advocate pressuring yourself at work, but for a career you love, it's worth the effort.
During my career, I often took time to follow the latest tech trends and fill my skill gaps.
Opportunities come to those who are prepared. I didn't wait to need a job to learn its required skills. Because I was interested, I prepared in advance, winning the chance to work those jobs.
This leads to today's last topic: How can I learn more effectively? My answer: actively practice.
Active Practice
There's a Chinese saying: "A good memory is not as good as a bad pen." Rather than relying on memory for everything you read, it's better to write summaries and solve problems.
If you're into Kong Fu, you know that just practicing a manual from start to finish is "by the book" learning. You need practical experience to handle various situations.
So, get involved in work to find your or your users' pain points, then learn everything to solve them.
If your job lacks practical opportunities, I recommend participating in projects outside of work.
Joining open-source projects is a great way to let more people see your work and help you improve. Or you could join Kaggle competitions, offering low-cost trial-and-error opportunities.
Or like me, start a blog like Data Leads Future to document your projects, summarize your progress, and help others.
Summary
Let's return to the beginning. How do you land your dream job without specific skills?
My answer: Start working hard now, keep loving and focusing, embrace change, continue learning, and actively practice.
Becoming a data scientist might seem amazing now, but over your career, it's just one milestone. Once you build enough strength, I believe you'll achieve greater success and make more contributions.