Unleashing the Power of Python Asyncio’s Queue
Mastering the producer-consumer pattern with asyncio through real-life examples
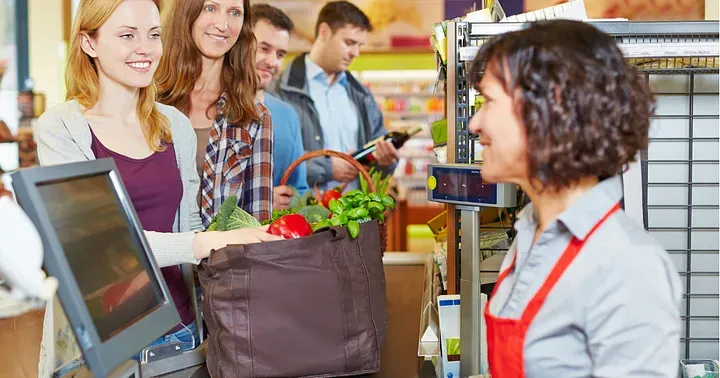
In this article, I will explain the API usage and application scenarios of various queues in Python asyncio relaxedly.
At the end of the article, I will demonstrate the practical usage of asyncio.Queue
in a classic shopping scenario.
Introduction
Why do we need asyncio.Queue
As readers who have read my previous articles know, I love asyncio because it is an almost perfect solution for concurrent programming.
However, in a large-scale, highly concurrent project, a large number of uncontrollable concurrent tasks waiting will occupy system resources, leading to poor performance.
Therefore, it is necessary to control the number of concurrent tasks.
Why can’t we use asyncio.Semaphore
In my previous article on synchronization primitives, I introduced using Semaphore
locks to control the number of concurrent tasks running simultaneously.
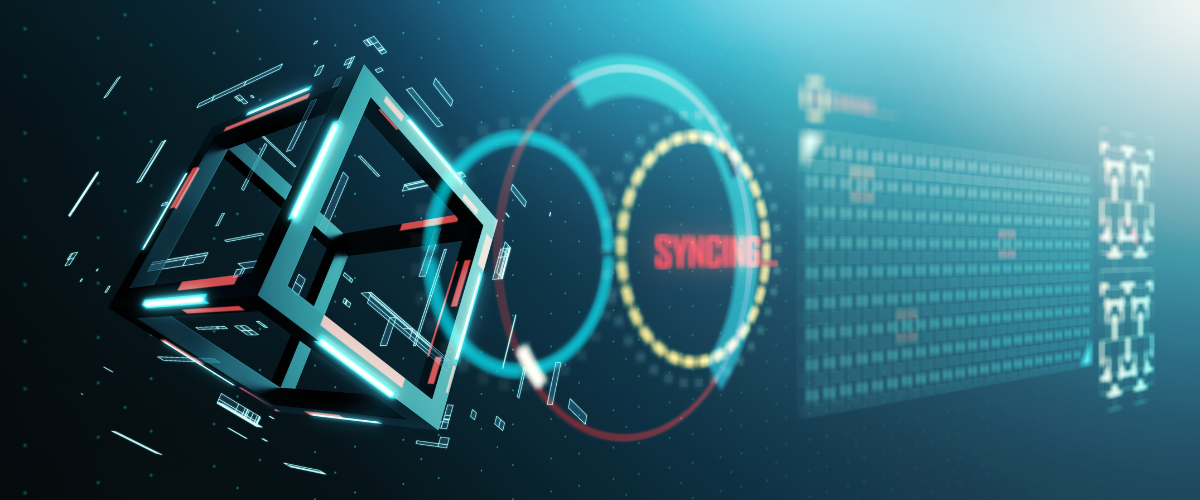
Set the number of Semaphore
locks first, and the tasks that get the lock will be executed, while those who don’t will wait.
However, asyncio.Semaphore
can only limit the concurrency of tasks accessing a resource through IO; the number of concurrent tasks cannot be limited.
Therefore, in this scenario, asyncio.Semaphore
is not a perfect solution.
asyncio.Queue is the way
Using asyncio.Queue
, we can start a fixed number of concurrent tasks when the program starts, and then pass the data to be processed through the queue to these tasks.
This is the well-known producer-consumer pattern. At the same time, like the multiprocessing queue, asyncio.Queue
also serves to pass messages between concurrent tasks.
The Magical World of asyncio’s Queue
Why can asyncio.Queue
plays such a significant role? In fact, we also encounter similar problems in real life:
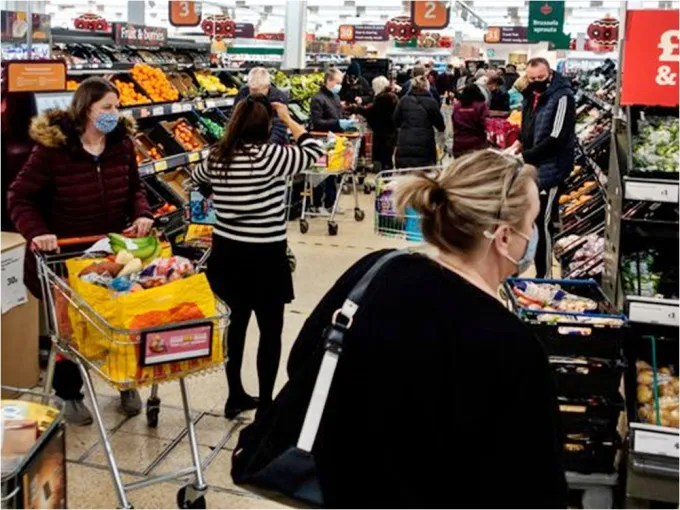
The most typical example is large shopping supermarkets. In such supermarkets, there are always many customers.
After each customer finishesshopping, they need to check out. Checking out takes some time, which can lead to congestion.
A more straightforward solution is to hire more cashiers, preferably one for each customer, for instant checkouts.
However, this is unrealistic because so many cashiers would mean colossal cost pressure (and resource consumption) for the boss.
So, a brilliant person came up with a good solution: have customers line up in a queue, and then have a few cashiers check out customers in turn.
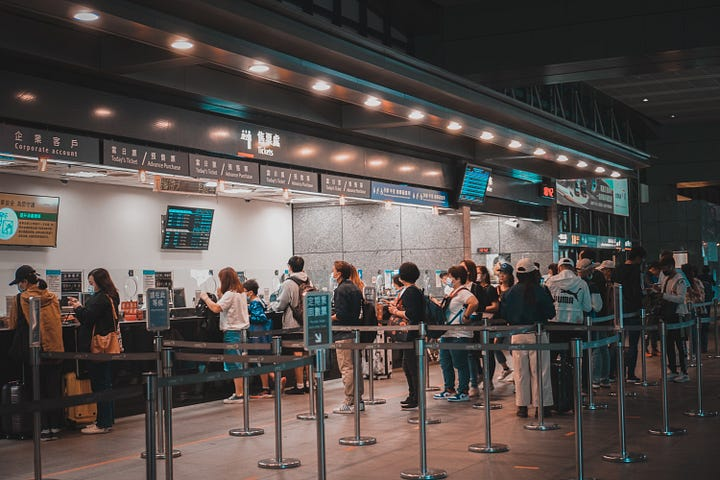
The only cost is that customers need to wait a little longer. At the same time, if the queue is too long, the manager can choose to temporarily add a few more cashiers. In this way, the system can flexibly expand.
By comparing the customer queue to data entering the queue and cashiers to concurrent tasks, we can see the benefits asyncio.Queue
brings:
- It is a good implementation of the producer-consumer pattern.
- It can control the number of concurrent tasks.
- Making resource consumption manageable, and the system can also be flexibly expanded.
The Adventures of the Producer-Consumer Pattern in asyncio
What is the producer-consumer pattern
Imagine two types of tasks sharing a queue. Task A produces data and puts it into the queue, while Task B retrieves data from the queue for processing.
This is the producer-consumer pattern, where Task A is the producer, and Task B is the consumer.
In analogy with a supermarket, customers are producers, cashiers are consumers, and the customer queue represents the queue.
Why use the producer-consumer pattern
In high-concurrency programs, producers often generate data quickly, while consumers process data slowly. Thus, producers must wait for consumers to finish processing before continuing to produce data.
Sometimes, consumers process data quickly, while producers generate data slowly. This leads to consumers waiting for producers to generate data before continuing to run.
To balance between producers and consumers, a queue is needed to store the data produced by the producer. The queue acts as a buffer and decouples the producer and consumer.
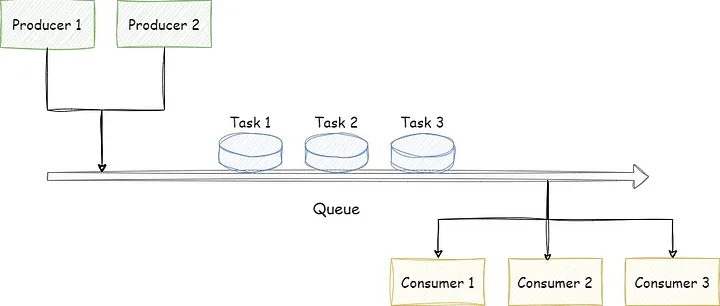
Implementing the Producer-Consumer Pattern with asyncio’s Queue
Now, let’s implement the supermarket shopping scenario mentioned earlier using asyncio.Queue
.
import asyncio
from asyncio import Queue
from random import randrange
class Product:
def __init__(self, product_name: str, checkout_time: float):
self.product_name = product_name
self.checkout_time = checkout_time
class Customer:
def __init__(self, customer_id: int, products: list[Product]):
self.customer_id = customer_id
self.products = products
As shown in the code, we first implement the Customer
and Product
classes, representing customers and products that need to be checked out. The Product
class has a checkout_time
attribute, which represents the time required for checking out the product.
After that, we implement a checkout_customer
method that acts as a consumer.
async def checkout_customer(queue: Queue, cashier_number: int):
while not queue.empty():
customer: Customer = await queue.get()
print(f"The Cashier_{cashier_number} "
f"will checkout Customer_{customer.customer_id}")
for product in customer.products:
print(f"The Cashier_{cashier_number} "
f"will checkout Customer_{customer.customer_id}'s "
f"Product_{product.product_name}")
await asyncio.sleep(product.checkout_time)
print(f"The Cashier_{cashier_number} "
f"finished checkout Customer_{customer.customer_id}")
queue.task_done()
As long as there is data in the queue, this method will continue to loop. During each iteration, it uses a get
method to retrieve a Customer
instance.
If there is no data in the queue, it will wait.
After retrieving a piece of data (in this case, a Customer
instance), it iterates through the products
attribute and uses asyncio.sleep
to simulate the checkout process.
After finishing processing the data, we use queue.task_done()
to tell the queue that the data has been successfully processed.
Next, we implement the generate_customer
method as a factory method for producing customers.
We first define a product series and the required checkout time for each product. Then, we place 0 to 10 products in each customer’s shopping cart.
def generate_customer(customer_id: int) -> Customer:
all_products = [Product('deer', 2),
Product('banana', .5),
Product('sausage', .2),
Product('diapers', .2)]
products = [all_products[randrange(len(all_products))] for _ in range(randrange(10))]
return Customer(customer_id, products)
Furthermore, we implement the customer_generation
method as a producer. This method generates several customer instances regularly and puts them in the queue. If the queue is full, the put
method will wait.
async def customer_generation(queue: Queue):
customer_count = 0
while True:
customers = [generate_customer(the_id)
for the_id in range(customer_count, customer_count+randrange(5))]
for customer in customers:
print("Waiting to put customer in line....")
await queue.put(customer)
print("Customer put in line...")
customer_count = customer_count + len(customers)
await asyncio.sleep(.3)
Finally, we use the main
method to initialize the queue, producer, and consumer, and start all concurrent tasks.
async def main():
customer_queue = Queue(2)
customer_producer = asyncio.create_task(customer_generation(customer_queue))
cashiers = [checkout_customer(customer_queue, i) for i in range(3)]
await asyncio.gather(customer_producer, *cashiers)
if __name__ == "__main__":
asyncio.run(main())
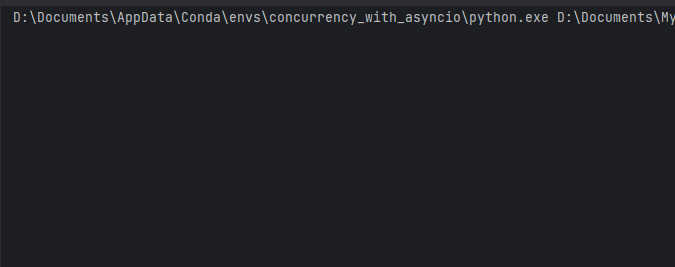
As expected, the implementation is successful.
Introducing the PriorityQueue
Why use asyncio.PriorityQueue
The queue mentioned earlier is a First-In-First-Out (FIFO) queue, where the first item to enter the queue is the first to be retrieved. This is suitable when all tasks in the queue have the same priority.
However, consider the following situation:
Suppose there is a queue with tasks waiting in line, each requiring a long processing time.
An error log or VIP user access is a high-priority task that needs immediate attention. What should we do?
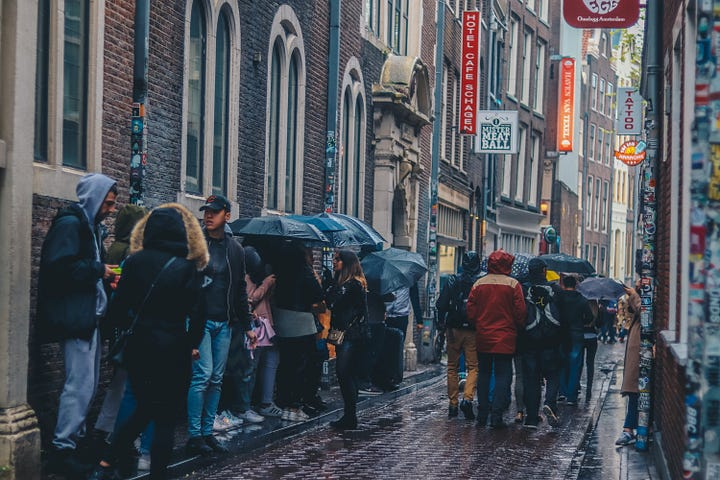
This is where asyncio.PriorityQueue
comes into play.
Briefly describe asyncio.PriorityQueue’s implementation
Unlike FIFO queues based on lists, asyncio.PriorityQueue
is based on heaps. It is built using a binary tree structure.
You may be familiar with binary search trees, which ensure that the most minor node is always the leftmost node.
However, the binary tree in asyncio.PriorityQueue
ensures that the most minor node is always at the top, so the highest priority node is permanently removed first.
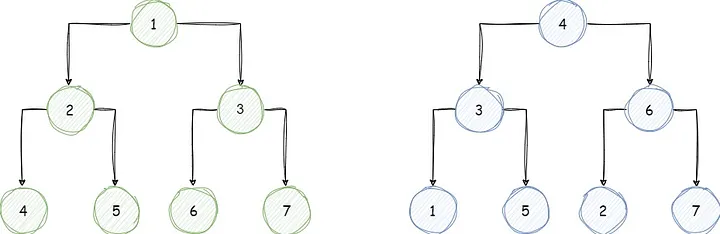
Real-world example with asyncio.PriorityQueue
Let’s illustrate the usage of asyncio.PriorityQueue
with a real-world scenario that exists in practice.
Imagine we have an order service API. The API takes time for each order to process, but we can’t keep users waiting too long.
So when a user places an order, the API first puts the order into a queue, allowing a background task to process it asynchronously while immediately returning a message to the user.
This API accepts orders from two types of users: regular users and VIP users. It must ensure that VIP user orders are processed with the highest priority.
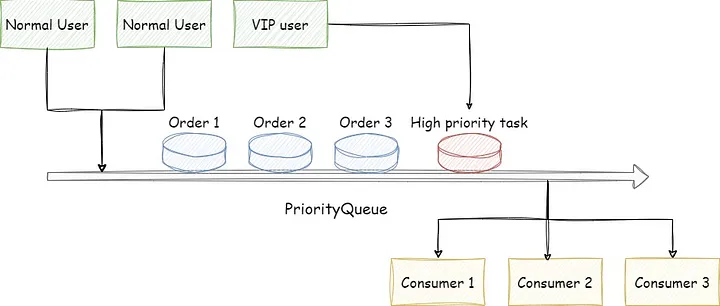
To keep the learning curve low for readers, in this example, we will use aiohttp
to implement the server. The specific code is as follows:
import asyncio
from asyncio import PriorityQueue, Task
from dataclasses import dataclass, field
from enum import IntEnum
from random import randrange
from aiohttp import web
from aiohttp.web_app import Application
from aiohttp.web_request import Request
from aiohttp.web_response import Response
app = Application()
routers = web.RouteTableDef()
QUEUE_KEY = "QUEUE_KEY"
TASK_KEY = "TASK_KEY"
class UserType(IntEnum):
POWER_USER = 1
NORMAL_USER = 2
@dataclass(order=True)
class WorkItem:
user_type: UserType
order_delay: int = field(compare=False)
First, we define an enumeration marking the two categories: regular users and VIP users.
Next, we use dataclass
to define a user's order, which contains the user type and order processing duration. The order duration is not considered in priority sorting.
Then we define the consumer method process_order_worker
, which retrieves orders from the queue and simulates the order processing.
Don’t forget to use queue.task_done()
to tell the queue that we finished processing the order.
async def process_order_worker(worker_id: int, queue: PriorityQueue):
while True:
work_item: WorkItem = await queue.get()
print(f"process_order_worker: Worker_{worker_id} begin to process worker {work_item}")
await asyncio.sleep(work_item.order_delay)
print(f"process_order_worker: Worker_{worker_id} finished to process worker {work_item}")
queue.task_done()
Following that, we implement the order API using aiohttp
. This API responds to user requests, generates an order object, and places it in the asyncio.PriorityQueue
.
It then immediately returns a response to the user, avoiding user wait time.
@routers.post("/order")
async def order(request: Request) -> Response:
queue: PriorityQueue = app[QUEUE_KEY]
body = await request.json()
user_type = UserType.POWER_USER if body['power_user'] == 'True' else UserType.NORMAL_USER
work_item = WorkItem(user_type, randrange(5))
await queue.put(work_item)
return Response(body="order placed!")
When the program starts, we use create_order_queue
to initialize the queue and order consumption tasks.
async def create_order_queue(app: Application):
print("create_order_queue: Begin to initialize queue and tasks.")
queue: PriorityQueue = PriorityQueue(10)
tasks = [asyncio.create_task(process_order_worker(i, queue)) for i in range(3)]
app[QUEUE_KEY] = queue
app[TASK_KEY] = tasks
print("create_order_queue: Initialize queue and tasks success..")
When the program ends, we use destroy_order_queue
to ensure that all orders in the queue are processed and the background tasks are closed correctly.
queue.join()
will wait for all the data in the queue to be processed. asyncio.wait_for
sets a timeout of 20 seconds, after which it will no longer wait queue.join()
to complete.
async def destroy_order_queue(app: Application):
queue: PriorityQueue = app[QUEUE_KEY]
tasks: list[Task] = app[TASK_KEY]
try:
print("destroy_order_queue: Wait for 20 sec to let all work done.")
await asyncio.wait_for(queue.join(), timeout=20.0)
except Exception as e:
print("destroy_order_queue: Cancel all tasks.")
[task.cancel() for task in tasks]
app.add_routes(routers)
app.on_startup.append(create_order_queue)
app.on_shutdown.append(destroy_order_queue)
web.run_app(app)
We can test this implementation using PyCharm’s HTTP Request:
POST http://localhost:8080/order
Content-Type: application/json
{"power_user": "True"}
###
POST http://localhost:8080/order
Content-Type: application/json
{"power_user": "False"}
###
POST http://localhost:8080/order
Content-Type: application/json
{"power_user": "False"}
###
POST http://localhost:8080/order
Content-Type: application/json
{"power_user": "True"}
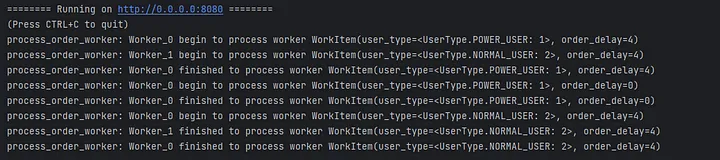
As you can see, the two high-priority tasks are processed as expected. Perfect!
Conclusion
In this article, I introduced the usage and best practices of asyncio.Queue
.
When you need to control the concurrency of a program, I recommend using asyncio.Queue
to effectively manage resource consumption.
I introduced the producer-consumer pattern and its benefits:
- Balancing between producers and consumers, maximizing resource utilization.
- Decoupling the system, allows producers and consumers to scale independently.
Finally, I showed how to use asyncio.PriorityQueue
to handle scenarios where tasks require prioritization through a real-world example.
Due to space constraints, I could not cover all aspects of asyncio.Queue
. However, I hope this article provided a solid understanding of the basic concepts and helpful examples.
Asynchronous programming in Python is a powerful tool, and the producer-consumer pattern with asyncio.Queue
is a versatile approach to handling concurrency and prioritization in your applications.